Intel recently collaborated with Novartis for using Deep Neural Networks (DNN). These DNNs are capable for accelerating high level content screening which is one of the most important parts of drug discovery. The DNNs reduced the train image analysis modelling time from 11 hours to half an hour which is a great improvement up to 20 times.
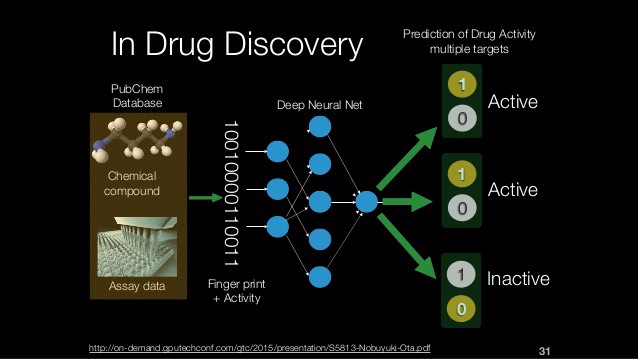
Fig.1 : Image Source: Deep Neural Network For Drug Discovery
For this purposes, Novartis and Intel team members used 8 CPU-based servers, an optimized TensorFlow and a high-speed fabric interconnect for attaining the much needed improvement for processing a dataset of 10, 000 images. So why is it so important? Cellular phenotypes high content screening is a basic tool highly favors early drug discovery. The phrase “high content” symbolizes a presence of hundreds and thousands of rich predefined set of features that are extracted from images with the help of classical image-processing techniques. Higher content screening enable microscopic images analysis for studying impact of thousand of chemical and genetic treatment on various cell cultures.
Deep learning here ensures that important image features that differentiate one kind of treatment from another are learned automatically from the data. So when a deep neural network is applied it helps data scientists and biologists at Novartis and Intel wish to pace up the high content imaging screens analysis. In this joint project, the team is currently emphasizing on microscopic image which is contrary to utilization of a separate procedure for identification of cell in a given image. Whole microscopy images could be quite larger in size than the ones are usually found in several deep learning datasets.
Deep convolutional neural network models that are used for microscopy images analysis typically act on million of pixels per image, it means it has millions and millions of parameters in model and probably thousands and thousands of training images in a go. This leads to heavy computational load. Even the most developed computational abilities on present day computers the deeper journey into DNN models might turn restrictive in terms of time.
Deep neural network acceleration technologies are being applied by the team for solving these challenges. These techniques would help in processing multiple images in much less time while going deeper into image features that are learned by the model ultimately.
Filed Under: News
Questions related to this article?
👉Ask and discuss on EDAboard.com and Electro-Tech-Online.com forums.
Tell Us What You Think!!
You must be logged in to post a comment.